Remember when Werner Vogels shared Amazonâs âworking backwardsâ (PR/FAQ) approach way back in 2006? Okay, me neither⊠but I think itâs fair to say that when Amazon does something well, itâs worth at least a bit of time to give it a look and see what works.
The PR/FAQ (stands for Press Release/Frequently Asked Questions) has been written about and opined on plenty, and Vogelâs original post I linked to above explains it best, so I wonât waste your time with it here. But at Tinybird, we use this approach with a twist: We write a BP(blog post)/FAQ, because do people even write press releases anymore?? Serious questionâŠ
Regardless of your disposition towards blogging or media glam, the point is pretty simple: When we build stuff, itâs best to think about the customer, whoever that may be, and cast a vision for how they will experience the end product before we start to iron out the technical wrinkles.
We take this approach when we build new features and products at Tinybird, and itâs also how we encourage our customers to build new data projects in Tinybird.
Let me explain.
A Tinybird data project consists of 2 basic components: Data Sources and Pipes (that often become API endpoints). We ingest data into Data Sources, transform it in Pipes with iterative SQL, and publish a node of the Pipe as an API endpoint in a single click. Boom đ. The process of creating an endpoint from raw data is super simple and takes minutes.
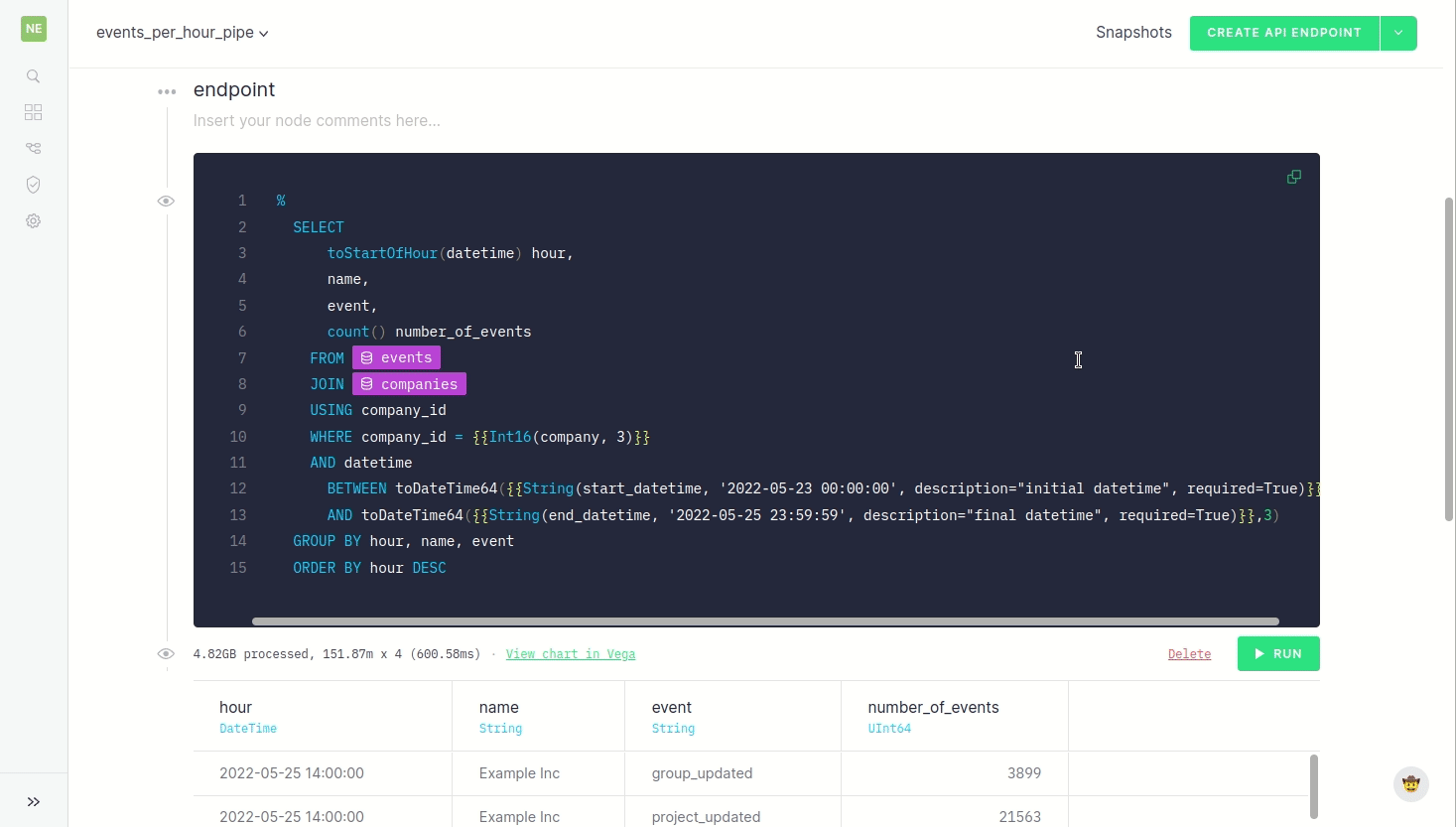
Of course, once that endpoint is created, there are almost always ways to make it better. How might we make it scan fewer rows? How might we shrink latency? These are important questions, but the process of optimizing endpoints can get pretty technical⊠We can start with pretty basic stuff like refactoring the schema and then we can really scrutinize and improve speed by creating materialized views (that is, Pipes that, instead of ending in an endpoint, end in a Data Source with the best schema and/or aggregation to make queries faster) or other important good practices like using subqueries for JOINs.
Start with a "minimally viable endpoint"
But in order to improve performance, you always need to have in mind what queries and endpoints youâre going to build. First create a prototype, then iterate to improve it. Only once youâve validated the use case with a prototype should you spend a lot of time choosing the correct indexes, tweaking your data types, and improving your queries.
So we always encourage our customers, when they first start with Tinybird, to get to the endpoint ASAP and worry about optimizations later. Why do we recommend this? Well, itâs easy to get hung up on the technical details too soon, and itâs really difficult to optimize something without knowing the desired end result. You risk losing sight of the ultimate value that Tinybird offers, which is building on top of data.
This is why Tinybird does stuff like recommend schema for your data sources or automatically choose an optimal configuration for (most) Materialized Views: so you can get to your results faster. We donât want you to obsess over your data source schemas or whether your pipes need to be materialized and then not build an endpoint. We want you to build endpoints so you can build products!
Of course, Iâm not suggesting that optimized endpoints and efficient data projects are frivolous pursuits. Quite the opposite. Our end goal is and always will be endpoints that serve highly concurrent, low-latency (ms), fresh results without scanning any more of the database than is necessary. But to get there, we think itâs really useful to build a âminimally viable endpointâ and then work backwards towards making that endpoint exceptional. Weâve built Tinybird to help you to do that.
A practical guide with a repo
If all of this seems pie in the sky, then maybe it is. But here are some practical steps to explain the process. If you want to follow along, I created a repo here that shows how you can build a minimally viable endpoint, and what kind of improvements you can get once you refactor. Iâve made commentary about the example in italics.
Start with a sample Data Source.
Rather than setting up ingestion pipelines from the beginning, start with a sample set of data. One of the simplest ways to do this is to drag and drop a CSV or NDJSON file into the Tinybird UI.
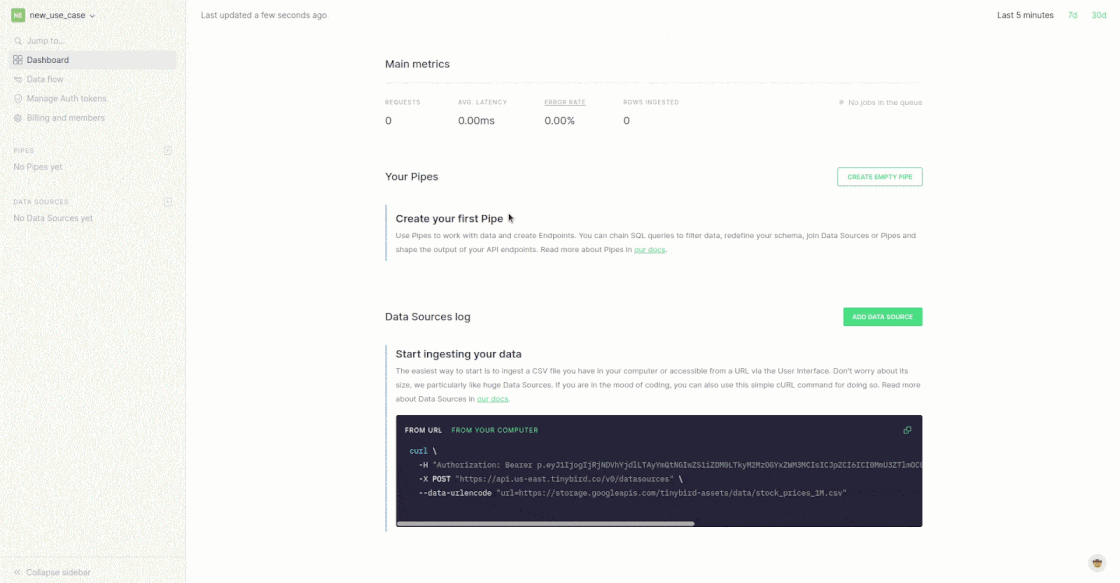
Note that when you set up this new Data Source, Tinybird automatically suggests a schema. Iâm gonna go out on a limb and ask you to trust us for now. Maybe that Int16 should be a UInt8, but weâll figure that out later. đ
For our example we are using our tried and true audit log demo: events generated by users while working with an app.
Define what you want your result to be.
Youâre not going to build an endpoint (yet), but you need to imagine what it will do. What kind of data will it serve? What needs to be parameterized? Answer these questions before you start building your Pipes.
In the example we want the endpoint to serve the number of events per hour, company, and type of event. And our users will want to filter by a date range and company when they call the endpoint.
Build a Pipe!
Ahhh, the fun part. Take that sample data and use SQL (following best practice, of course) to iteratively transform the data towards your endpoint.
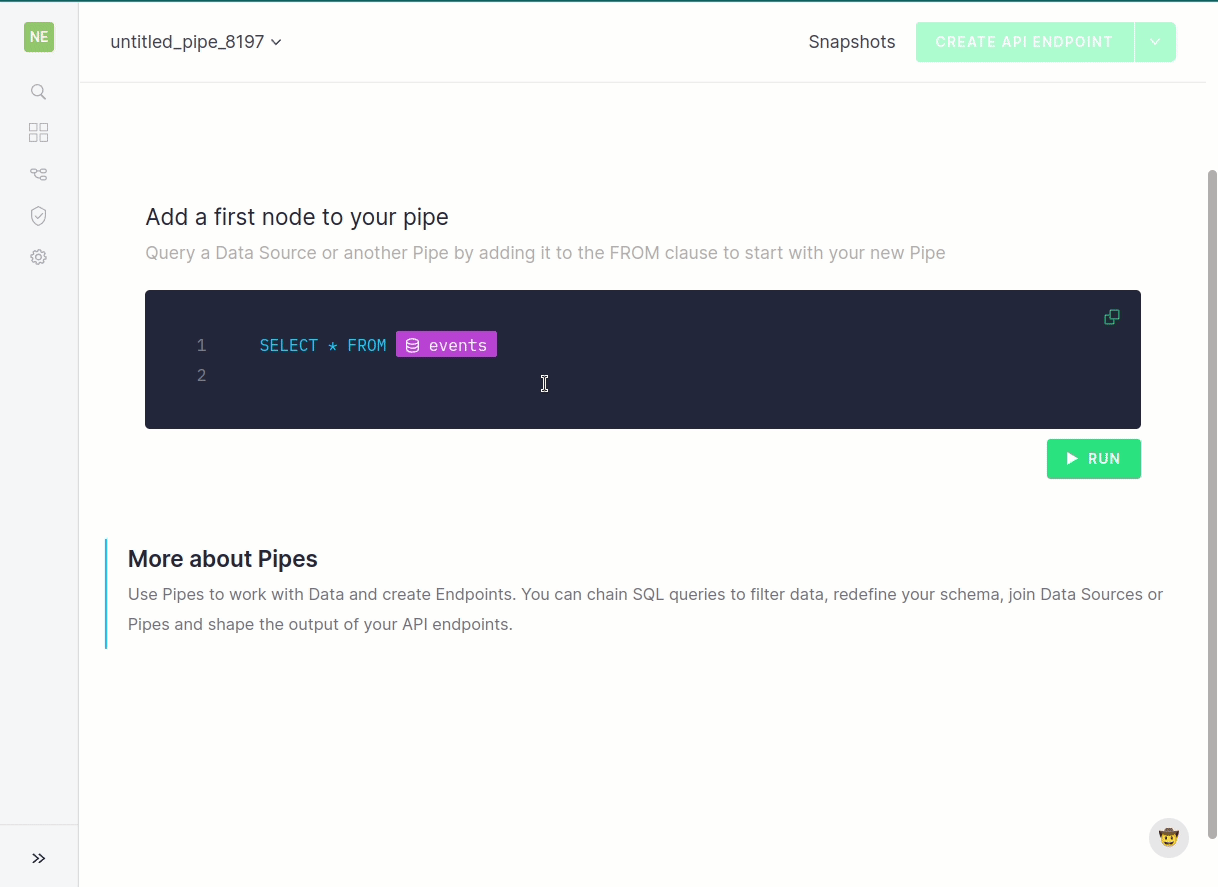
Hopefully youâre able to get to a point where your endpoint effectively delivers the result you defined previously, even if performance isnât optimal.
This is a good place to stop working in Tinybird (if only temporarily), and start building product prototypes on top of your new endpoint. Youâll get a very good feel for how the endpoints youâve created serve your application, and if you do need to make tweaks to your pipes or add a new parameter, you can do that here.
Alternatively, you might discover that your original data source didnât work out as planned. Maybe you donât have what you need to effectively generate your desired result. And this is precisely why we encourage you to work this way: Itâs a real buzzkill to spend hours ingesting all your data or thinking about the best queries and schema only to realize your data source needs to be tweaked to serve the use case.
Finally, refactor.
Tinybird does its best to choose the right schema for your data sources, but weâre not precogs here. We canât predict every use case. But now that youâve created endpoints and taken them for a spin, you should have a pretty good idea of how you want to use them, and how you can refactor your schemas to make them blazing fast.
Hereâs what you can do to find big gains in this stage, first with Data Source operations:
- Adjust your data types. In particular, focus on converting Nullables to something else (coalesce '', 0âŠ), downsizing types (UInt instead of Int when negatives arenât possible), and checking String cardinality (LowCardinality types offer savings over String).
- Change your indexes. Your indexes define how data is stored in the table, and properly-defined indexes make lookups way faster. Remember the importance of sequential reads!â
- âCheck your partition key. Changing the partition key wonât necessarily improve speed, but a badly defined partition can cause problems later. Here are some tips for partition keys.
Back to our example: we reduced the data types by using LowCardinality, and, most importantly, edited our indexes to match our final use case. We always filter by company and time range, so we should use these as indexes. You can see here how changing the schema made a huge improvement in the speed and amount of processed data. Same result, just faster.
⊠then with some other, more advanced improvements:
- Use Materialized Views. Materialized Views are basically the antimatter of Tinybird data projects, and they have the potential to massively reduce latency and scan size of your endpoints. If your Pipes always perform the same aggregations or transformations, Materialized Views are a perfect fit here.
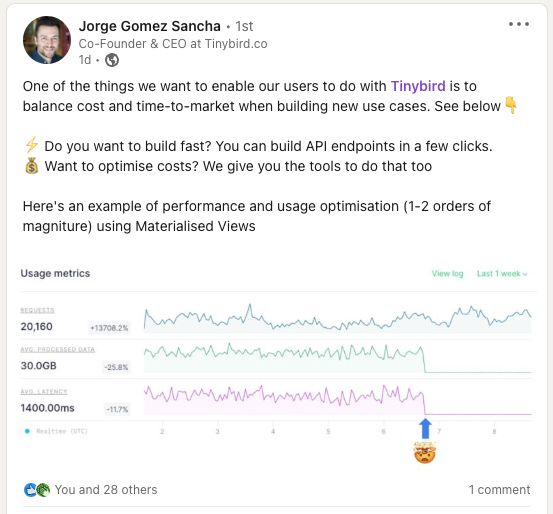
- Consider subqueries for your JOINs. If youâre using a plain JOIN and the table that youâre joining grows, itâs going to slow down your query. Use a subquery to only JOIN on the data you need. Hereâs an example that our friends at Typeform recently wrote about on our blog.
I hope this helps explain why we think the âworking backwardsâ attitude works for product development. The important point is this: Tinybird was built for speed. That means speed in our product (aka blazing endpoint response times), but also speed when building the product (through rapid prototyping and iteration of the data project). âSpeed wins'' is our de facto company motto. We even put it on socksâŠ
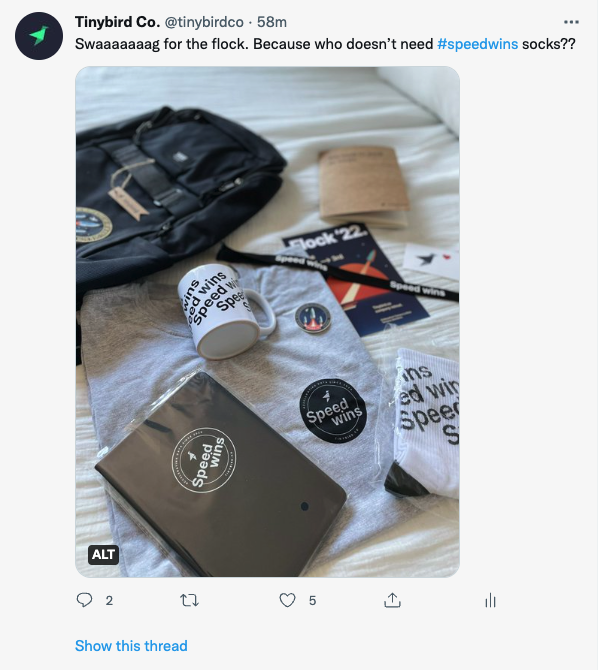
p.s. If you have any questions about this (or maybe you just want some of that đ„ swag ), then feel free to join our community Slack channel and DM me.